Towards Greater (Local) Relevance of Causal Generalizations
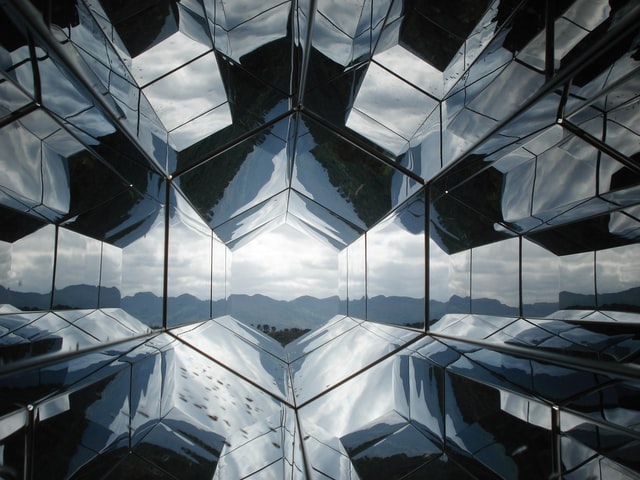
To cite the paper we discuss in this blog post, use the reference below.
Jaciw, A. P., Unlu, F., & Nguyen, T. (2021). A within-study approach to evaluating the role of moderators of impact in limiting generalizations from “large to small”. American Journal of Evaluation. https://journals.sagepub.com/doi/10.1177/10982140211030552
Generalizability of Causal Inferences
The field of education has made much progress over the past 20 years in the use of rigorous methods, such as randomized experiments, for evaluating causal impacts of programs. This includes a growing number of studies on the generalizability of causal inferences stemming from the recognition of the prevalence of impact heterogeneity and its sources (Steiner et al., 2019). Most recent work on generalizability of causal inferences has focused on inferences from “small to large”. Studies typically include 30–70 schools while generalizations are made to inference populations at least ten times larger (Tipton et al., 2017). Such studies are typically used in informing decision makers concerned with impacts on broad scales, for example at the state level. However, as we are periodically reminded by the likes of Cronbach (1975, 1982) and Shadish et al. (2002), generalizations are of many types and support decisions on different levels. Causal inferences may be generalized not only to populations outside the study sample or to larger populations, but also to subgroups within the study sample and to smaller groups – even down to the individual! In practice, district and school officials who need local interpretations of the evidence might ask: “If a school reform effort demonstrates positive impact on some large scale, should I, as a principal, expect that the reform will have positive impact on the students in my school?” Our work introduces a new approach (or a new application of an old approach) to address questions of this type. We empirically evaluate how well causal inferences that are drawn on the large scale generalize to smaller scales.
The Research Method
We adapt a method from studies traditionally used (first in economics and then in education) to empirically measure the accuracy of program impact estimates from non-experiments. A central question is whether specific strategies result in better alignment between non-experimental impact findings and experimental benchmarks. Those studies—sometimes referred to as “Within-Study Comparison” studies (pioneered by Lalonde, 1986, and Fraker et al., 1987)—typically start with an estimate of a program’s impact from an uncompromised experiment. This result serves as the benchmark experimental impact finding. Then, to generate a non-experimental result, outcomes from the experimental control are replaced with those from a different comparison group. The difference in impact that results from this substitution measures the bias (inaccuracy) in the result that employs the non-experimental comparison. Researchers typically summarize this bias, and then try to remediate using various design and analysis-based strategies. (The Within-Study Comparison literature is vast and includes many studies that we cite in the article.)
Our Approach Follows a Within-Study Comparison Rationale and Method, but with a Focus on Generalizability.
We use data from the multisite Tennessee Student-Teacher Achievement Ratio (STAR) class size reduction experiment (described in Finn et al., 1990; Mosteller, 1995; Nye et al., 2000) to illustrate the application of our method. (We used 73 of the original 79 sites.) In the original study, students and teachers were randomized to small or regular-sized classes in grades K-3. Results showed a positive average impact of small classes. In our study, we ask whether a decisionmaker at a given site should accept this finding of an overall average positive impact as generalizable to his/her individual site.
We use the Within-Study Comparison Method as a Foundation.
First, we adopt the idea of using experimental benchmark impacts as the starting point. In the case of the STAR trial, each of the 73 individual sites yields its own benchmark value for impact. Second, consistent with Within-Study Comparisons, we select an alternative to compare against the benchmark. Specifically, we choose the average of impacts (the grand mean) across all sites as the generalized value. Third, we establish how closely this generalized value approximates impacts at individual sites (i.e., how well it generalizes “to the small”.) With STAR, we can do this 73 times, once for each site. Fourth, we summarize the discrepancies. Standard Within-Study Comparison methods typically average over the absolute values of individual biases. We adapt this, but instead use the average of 73 squared differences between the generalized impact and site-benchmark impacts. This allows us to capture the average discrepancy as a variance, specifically as the variation in impact across sites. We estimated this variation several ways, using alternative hierarchical linear models. Finally, we examine whether adjusting for imbalance between sites in site-level characteristics that potentially interact with treatment leads to closer alignment between the grand mean (generalized) and site-specific impacts. (Sometimes people wonder why, with Within-Study Comparison studies, if site-specific benchmark impacts are available, one would use less-optimal comparison group-based alternatives. With Within-Study Comparisons, the whole point is to see how closely we can replicate the benchmark quantity, in order to inform how well methods of causal inference (of generalization, in this case) potentially perform, in situations where we do not have an experimental benchmark.)
Our application is intentionally based on Within-Study Comparison methods. This is set out clearly in Jaciw (2010, 2016). Early applications with a similar approach can be found in Hotz, et al. (2005) and Hotz, et al. (2006). A new contribution of ours is that we summarize the discrepancy not as an average of absolute value of bias (a common metric in Within-Study Comparison studies), but as noted above, as a variance. This may sound like a nuanced technical detail, but we think it leads to an important interpretation: variation in impact is not just related to the problem of generalizability; rather, it directly indexes the accuracy (quantifies the degree of validity) of generalizations from “large to small”. We acknowledge Bloom et al. (2005) for the impetus for this idea, specifically, their insight that bias in Within-Study Comparison studies can be thought of as a type of “mismatch error”. Finally, we think it is important to acknowledge the ideas in G Theory from education (Cronbach et al., 1963; Shavelson et al., 2009). In that tradition, parsing variability in outcomes, accounting for its sources, and assessing the role of interactions among study factors, are central to the problem of generalizability.
Research Findings
First main result
The grand mean impact, on average, does not generalize reliably to the 73 sites. Before covariate adjustments, the average of the differences between the grand mean and the impacts at individual sites ranges between 0.41 and 0.25 standard deviations (SDs) of the outcome distribution, depending on the model used. After covariate adjustments, the average of the differences ranges between 0.41 and 0.17 SDs. (The average impact was about 0.25 SD.)
Second main result
Modeling effects of site-level covariates, and their interactions with treatment, only minimally reduced the between-site differences in impact.
The third main result
Whether impact heterogeneity achieves statistical significance depends on sampling error and correctly accounting for its sources. If we are going to provide accurate policy advice, we must make sure that we are not confusing random sampling error within sites (differences we would expect in results even if the program was not used) for variation in impact across sites. One source of random sampling error that is important but could be overlooked comes from classes. Given that teachers provide different value-added to students’ learning, we can expect differences in outcomes across classes. In STAR, with only a handful of teachers per school, the between-class differences easily add noise to the between-school outcomes and impacts. After adjusting for class random effects, the discrepancies in impact described above decreased by approximately 40%.
Research Conclusions
For the STAR experiment, the grand mean impact failed to generalize to individual sites. Adjusting for effects of moderators did not help much. Adjusting for class-level sampling error significantly reduced the level of measured heterogeneity. Even though the discrepancies decreased significantly after the class effects were included, the size of the discrepancies remained large enough to be substantively important, and therefore, we cannot conclude that the average impact generalized to individual sites.
In sum, based on this study, a policymaker at the site (school) level should apply caution in assessing whether the average result applies to his or her unique context.
The results remind us of an observation from Lee Cronbach (1982) about how a school board might best draw inferences about their local context serving a large Hispanic student body when program effects vary:
The school board might therefore do better to look at…small cities, cities with a large Hispanic minority, cities with well-trained teachers, and so on. Several interpretations-by-analogy can then be made….If these several conclusions are not too discordant, the board can have some confidence in the decision that it makes about its small city with well-trained teachers and a Hispanic clientele. When results in the various slices of data are dissimilar, it is better to try to understand the variation than to take the well-determined – but only remotely relevant – national average as the best available information. The school board cannot regard that average as superior information unless it believes that district characteristics do not matter (p. 167).
Some Possible Extensions of The Work
We’re looking forward to doing more work to continue to understand how to produce useful generalizations that support decision-making on smaller scales. Traditional Within-Study Comparison studies give us much food for thought, including about other designs and analysis strategies for inferring impacts to individual sites, and how to best communicate the discrepancies we observe and whether they are substantively large enough to matter for informing policy decisions and outcomes. One area of main interest concerns the quality of the moderators themselves; that is, how well they account for or explain impact heterogeneity. Here our approach diverges from traditional Within-Study Comparison studies. When applied to problems of internal validity, confounders can be seen as nuisances that make our impact estimates inaccurate. With regard to external validity, factors that interact with the treatment, and thereby produce variation in impact that affects generalizability, are not a nuisance; rather, they are an important source of information that may help us to understand the mechanisms through which the variation in impact occurs. Therefore, understanding the mechanisms relating the person, the program, context, and the outcome is key.
Lee Cronbach described the bounty of and interrelations among interactions in the social sciences as a “hall of mirrors”. We’re looking forward to continuing the careful journey along that hall to incrementally make sense of a complex world!
References
Bloom, H. S., Michalopoulos, C., & Hill, C. J. (2005). Using experiments to assess nonexperimental comparison -group methods for measuring program effect. In H. S. Bloom (Ed.), Learning more from social experiments (pp. 173 –235). Russell Sage Foundation.
Cronbach, L. J. (1975). Beyond the two disciplines of scientific psychology. American Psychologist, 30(2), 116–127.
Cronbach, L.J., Rajaratnam, N., & Gleser, G.C. (1963). Theory of generalizability: A liberation of reliability theory. The British Journal of Statistical Psychology, 16, 137-163.
Cronbach, L. J. (1982). Designing Evaluations of Educational and Social Programs. Jossey-Bass.
Finn, J. D., & Achilles, C. M., (1990). Answers and questions about class size: A statewide experiment. American Educational Research Journal, 27, 557-577.
Fraker, T., & Maynard, R. (1987). The adequacy of comparison group designs for evaluations of employment-related programs. The Journal of Human Resources, 22, 194–227.
Jaciw, A. P. (2010). Challenges to drawing generalized causal inferences in educational research: Methodological and philosophical considerations. [Doctoral dissertation, Stanford University.]
Jaciw, A. P. (2016). Assessing the accuracy of generalized inferences from comparison group studies using a within-study comparison approach: The methodology. Evaluation Review, 40, 199-240. https://journals.sagepub.com/doi/abs/10.1177/0193841x16664456
Hotz, V. J., Imbens, G. W., & Klerman, J. A. (2006). Evaluating the differential effects of alternative welfare-to-work training components: A reanalysis of the California GAIN Program. Journal of Labor Economics, 24, 521–566.
Hotz, V. J., Imbens, G. W. & Mortimer, J. H (2005). Predicting the efficacy of future training programs using past experiences at other locations. Journal of Econometrics, 125, 241–270.
Lalonde, R. (1986). Evaluating the econometric evaluations of training programs with experimental data. The American Economic Review, 76, 604–620.
Mosteller, F., (1995). The Tennessee study of class size in the early school grades. The Future of Children, 5, 113-127.
Nye, B., Hedges, L. V., & Konstantopoulos, (2000). The effects of small classes on academic achievement: The results of the Tennessee class size experiment. American Educational Research Journal, 37, 123-151.
Fraker, T., & Maynard, R. (1987). The adequacy of comparison group designs for evaluations of employment-related programs. The Journal of Human Resources, 22, 194–227.
Shadish, W. R., Cook, T. D., & Campbell, D. T., (2002). Experimental and Quasi-experimental Designs for Generalized Causal Inference. Houghton Mifflin.
Shavelson, R. J., & Webb, N. M. (2009). Generalizability theory and its contributions to the discussion of the generalizability of research findings. In K. Ercikan & W. M. Roth (Eds.), Generalizing from educational research (pp. 13–32). Routledge.
Steiner, P. M., Wong, V. C. & Anglin, K. (2019). A causal replication framework for designing and assessing replication efforts. Zeitschrift fur Psychologie, 227, 280–292.
Tipton, E., Hallberg, K., Hedges, L. V., & Chan, W. (2017). Implications of small samples for generalization: Adjustments and rules of thumb. Evaluation Review, 41(5), 472–505.
Jaciw, A. P., Unlu, F., & Nguyen, T. (2021). A within-study approach to evaluating the role of moderators of impact in limiting generalizations from “large to small”. American Journal of Evaluation. https://journals.sagepub.com/doi/10.1177/10982140211030552
Photo by drmakete lab