SREE 2022 Annual Meeting
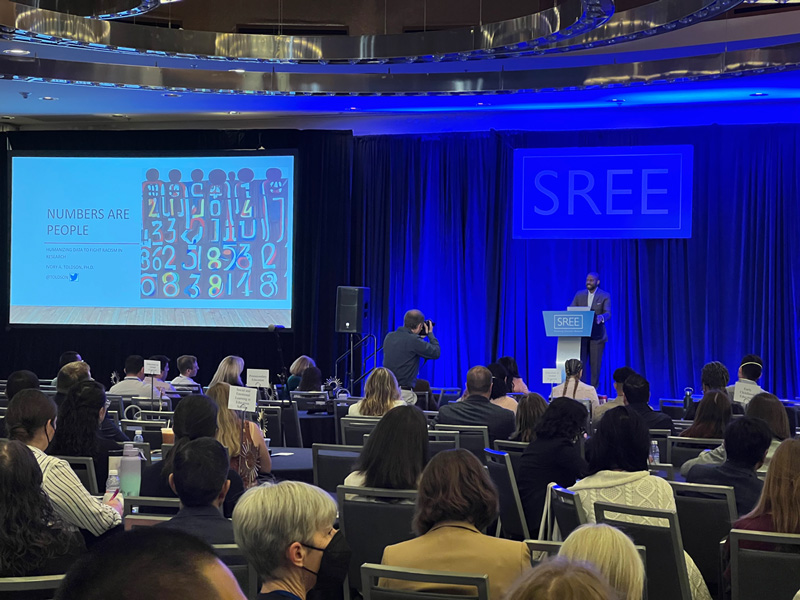
When I read the theme of the 2022 SREE Conference, “Reckoning to Racial Justice: Centering Underserved Communities in Research on Educational Effectiveness”, I was eager to learn more about the important work happening in our community. The conference made it clear that SREE researchers are becoming increasingly aware of the need to swap individual-level variables for system-level variables that better characterize issues of systematic access and privilege. I was also excited that many SREE researchers are pulling from the fields of mixed methods and critical race theory to foster more equity-aligned study designs, such as those that center participant voice and elevate counter-narratives.
I’m excited to share a few highlights from each day of the conference.
Wednesday, September 21, 2022
Dr. Kamilah B. Legette, University of Denver
Dr. Kamilah B. Legette from the University of Denver discussed their research exploring the relationship between a student’s race and teacher perceptions of the student’s behavior as a) severe, b) inappropriate, and c) indicative of patterned behavior. In their study, 22 teachers were asked to read vignettes describing non-compliant student behaviors (e.g., disrupting storytime) where student identity was varied by using names that are stereotypically gendered and Black (e.g., Jazmine, Darnell) or White (e.g., Katie, Cody).
Multilevel modeling revealed that while student race did not predict teacher perceptions of behavior as severe, inappropriate, or patterned, students’ race was a moderator of the strength of the relationship between teachers’ emotions and perceptions of severe and patterned behavior. Specifically, the relationship between feelings of frustration and severe behavior was stronger for Black children than for White children, and the relationship between feelings of anger and patterned behavior showed the same pattern. Dr. Legette’s work highlighted a need for teachers to engage in reflective practices to unpack these biases.
Dr. Johari Harris, University of Virginia
In the same session, Dr. Johari Harris from the University of Virginia shared their work with the Children’s Defense Fund Freedom Schools. Learning for All (LFA), one Freedom School for students in grades 3-5, offers a five-week virtual summer literacy program with a culturally responsive curriculum based on developmental science. The program aims to create humanizing spaces that (re)define and (re)affirm Black students’ racial-ethnic identities, while also increasing students’ literacy skills, motivation, and engagement.
Dr. Harris’s mixed methods research found that students felt LFA promoted equity and inclusion, and reported greater participation, relevance, and enjoyment within LFA compared to in-person learning environments prior to COVID-19. They also felt their teachers were culturally engaging, and reported a greater sense of belonging, desire to learn, and enjoyment.
While it’s often assumed that young children of color are not fully aware of their racial-ethnic identity or how it is situated within a White supremacist society, Dr. Harris’s work demonstrated the importance of offering culturally affirming spaces to upper-elementary aged students.
Thursday, September 22, 2022
Dr. Krystal Thomas, SRI
On Thursday, I attended a talk by Dr. Krystal Thomas from SRI International about the potential of open education resource (OER) programming to further culturally responsive and sustaining practices (CRSP). Their team developed a rubric to analyze OER programming, including materials and professional development (PD) opportunities. The rubric combined principles of OER (free and open access to materials, student-generated knowledge) and CRSP (critical consciousness, student agency, student ownership, inclusive content, classroom culture, and high academic standards).
Findings suggest that while OER offers access to quality instructional materials, it does not necessarily develop teacher capacity to employ CRSP. The team also found that some OER developers charge for CRSP PD, which undermines a primary goal of OER (i.e., open access). One opportunity this talk provided was eventual access to a rubric to analyze critical consciousness in program materials and professional learning (Dr. Thomas said these materials will be posted on the SRI website in upcoming months). I believe this rubric may support equity-driven research and evaluation, including Empirical’s evaluation of the antiracist teacher residency program, CREATE (Collaboration and Reflection to Enhance Atlanta Teacher Effectiveness).
Dr. Rekha Balu, Urban Institute; Dr. Sean Reardon, Stanford University; Dr. Beth Boulay, Abt Associates
The plenary talk, featuring discussants Dr. Rekha Balu, Dr. Sean Reardon, and Dr. Beth Boulay, offered suggestions for designing equity- and action-driven effectiveness studies. Dr. Balu urged the SREE community to undertake “projects of a lifetime”. These are long-haul initiatives that push for structural change in search of racial justice. Dr. Balu argued that we could move away from typical thinking about race as a “control variable”, towards thinking about race as an experience, a system, and a structure.
Dr. Balu noted the necessity of mixed methods and participant-driven approaches to serve this goal. Along these same lines, Dr. Reardon felt we need to consider system-level inputs (e.g., school funding) and system-level outputs (e.g., rate of high school graduation) in order to understand disparities in opportunity, rather than just focusing on individual-level factors (e.g., teacher effectiveness, student GPA, parent involvement) that distract from larger forces of inequity. Dr. Boulay noted the importance of causal evidence to persuade key gatekeepers to pursue equity initiatives and called for more high quality measures to serve that goal.
Friday, September 23, 2022
The tone of the conference on Friday was to call people in (a phrase used in opposition to “call people out”, which is often ego-driven, alienating, and counter-productive to motivating change).
Dr. Ivory Toldson, Howard University
In the morning, I attended the Keynote Session by Dr. Ivory Toldson from Howard University. What stuck with me from Dr. Toldson’s talk was their argument that we tend to use numbers as a proxy for people in statistical models, but to avoid some of the racism inherent in our profession as researchers, we must see numbers as people. Dr. Toldson urged the audience to use people to understand numbers, not numbers to understand people. In other words, by deriving a statistical outcome, we do not necessarily know more about the people we study. However, we are equipped with a conversation starter. For example, if Dr. Toldson hadn’t invited Black boys to voice their own experience of why they sometimes struggle in school, they may have never drawn a potential link between sleep deprivation and ADHD diagnosis: a huge departure from the traditional deficit narrative surrounding Black boys in school.
Dr. Toldson also challenged us to consider what our choice in the reference group means in real terms. When we use White students as the reference group, we normalize Whiteness and we normalize groups with the most power. This impacts not only the conclusions we draw, but also the larger framework in which we operate (i.e., White = standard, good, normal).
I also appreciated Dr. Toldson’s commentary on the need for “distributive trust” in schools. They questioned why the people furthest from the students (e.g., superintendents, principals) are given the most power to name best practices, rather than empowering teachers to do what they know works best and to report back. This thought led me to wonder, what can we do as researchers to lend power to teachers and students? Not in a performative way, but in a way that improves our research by honoring their beliefs and first-hand experiences; how can we engage them as knowledgeable partners who should be driving the narrative of effectiveness work?
Dr. Deborah Lindo, Dr. Karin Lange, Adam Smith, EF+Math Program; Jenny Bradbury, Digital Promise; Jeanette Franklin, New York City DOE
Later in the day, I attended a session about building research programs on a foundation of equity. Folks from EF+Math Program (Dr. Deborah Lindo, Dr. Karin Lange, and Dr. Adam Smith), Digital Promise (Jenny Bradbury), and the New York City DOE (Jeanette Franklin) introduced us to some ideas for implementing inclusive research, including a) fostering participant ownership of research initiatives; b) valuing participant expertise in research design; c) co-designing research in partnership with communities and participants; d) elevating participant voice, experiential data, and other non-traditional effectiveness data (e.g., “street data”); and e) putting relationships before research design and outcomes. As the panel noted, racism and inequity are products of design and can be redesigned. More equitable research practices can be one way of doing that.
Saturday, September 24, 2022
Dr. Andrew Jaciw, Empirical Education
On Saturday, I sat in on a session that included a talk given by my colleague Dr. Andrew Jaciw. Instead of relaying my own interpretation of Andrew’s ideas and the values they bring to the SREE community, I’ll just note that he will summarize the ideas and insights from his talk and subsequent discussion in an upcoming blog. Keep your eyes open for that!
See you next year!